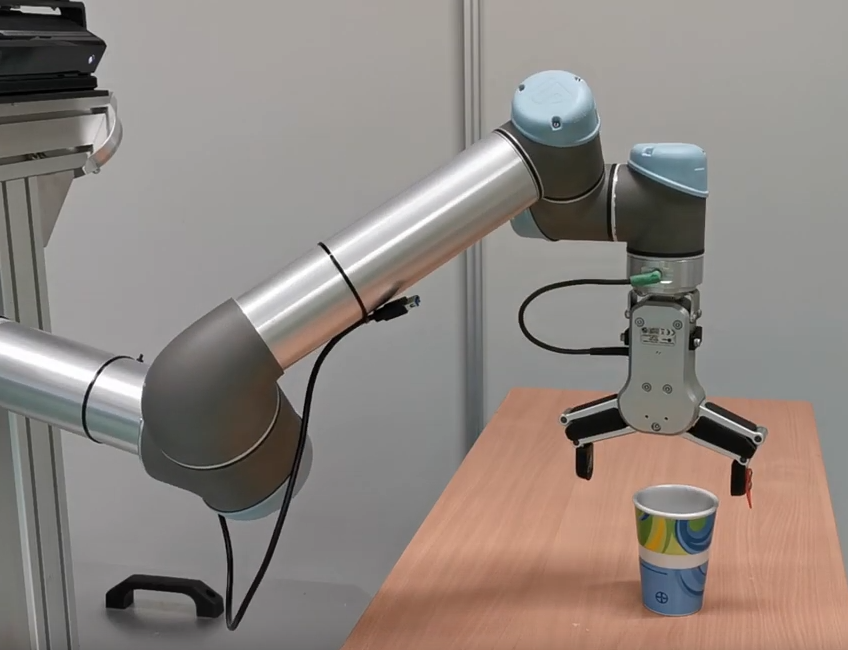
Robots face challenges in perceiving new scenes, particularly when registering objects from a single perspective, resulting in incomplete shape information about objects. Partial object models negatively influence the performance of grasping methods. To address this, robots can scan the scene from various perspectives or employ methods to directly fill in unknown regions. This research reexamines scene reconstruction typically formulated in 3D space, proposing a novel formulation in 2D image space for robots with RGB-D cameras. We introduce a method that generates a depth image from a virtual camera pose located on the opposite position of the reconstructed object. The article demonstrates that the convolutional neural network can be trained for accurate depth image generation and subsequent 3D scene reconstruction from a single viewpoint. We show that the proposed approach is computationally efficient and accurate when compared to methods that operate directly in 3D space. Furthermore, we illustrate the application of this model in enhancing grasping method success rates.
Rafał Staszak, Bartłomiej Kulecki, Marek Kraft, Dominik Belter, MirrorNet: Hallucinating 2.5D Depth Images for Efficient 3D Scene Reconstruction, Robotics and Autonomous Systems Robotics and Autonomous Systems, 2024